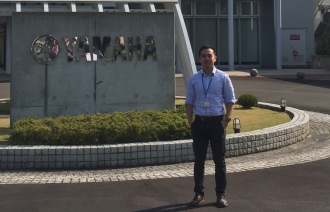
During Oct-Dec 2015, I was a research intern at Yamaha Corporation Japan supervised by Dr. Akira Maezawa for a sung melody transcription project. We employed the novel observation probability distribution of combination of angular and gamma distributions to the HMM-based framework. The work is accepted as a proceeding of the 42nd IEEE International Conference on Acoustics, Speech, and Signal Processing (ICASSP2017).

Akira Maezawa, Researcher of Music Information Retrieval and Statistical Audio Signal Processing at Yamaha Corporation Japan, presented our paper/poster at the 42nd IEEE International Conference on Acoustics, Speech, and Signal Processing in New Orleans, 5-9 March 2017, in the Pitch and Musical Analysis Session on Monday, March 6, 16:00-18:00. The other authors are Dr. Jordan B. L. Smith and Prof. Elaine Chew.
Reference:
Yang, L., A. Maezawa, J. B. L. Smith, E. Chew (2017). Towards pitch dynamic model based probabilistic transcription of sung melody. In Proceedings of the 42nd IEEE Conference on Acoustics, Speech, and Signal Processing (ICASSP), pp. 301-305, New Orleans, Mar 5-9, 2017. [ PDF ]
Abstract: Transcribing the singing voice into music notes is challenging due to pitch fluctuations such as portamenti and vibratos. This paper presents a probabilistic transcription method for monophonic sung melodies that explicitly accounts for these local pitch fluctuations. In the hierarchical Hidden Markov Model (HMM), an upper-level ergodic HMM handles the transitions between notes, and a lower-level left-to-right HMM handles the intra- and inter-note pitch fluctuations. The lower-level HMM employs the pitch dynamic model, which explicitly expresses the pitch curve characteristics as the observation likelihood over f_0 and Delta f_0 using a compact parametric distribution. A histogram-based tuning frequency estimation method, and some post-processing heuristics to separate merged notes and to allocate spuriously detected short notes, improve the note recognition performance. With model parameters that support intuitions about singing behavior, the proposed method obtained encouraging results when evaluated on a published monophonic sung melody dataset, and compared with state-of-the-art methods.